From a linear to a circular economy
In a linear economy, we use raw materials to manufacture a product, which we throw away after use. To evolve from such a linear to a more circular system, we need to transform every step: the way we handle natural resources, design, produce and use products, as well as what we do with the materials afterwards.
We are shifting the focus from waste management to material stock management. This allows us to push value retention strategies such as reuse, repair and refurbish, and significantly reduce the use of raw materials.
Yoko Dams, R&D expert circular materials management: "The construction sector and the built environment are the largest material consumers worldwide and represent the highest share of our Flemish waste production. In addition, the built environment has a significant impact on our climate and environment. In a circular economy, we regard building materials in the city as an “urban mine” for secondary materials, which benefits all stakeholders: citizens, industry and the livability and sustainability of our cities and municipalities. In the Demolition Guide project we have been evaluating how we can use data science to support or even accelerate this transition."
Foremost, we need detailed information about the stock of materials present in the built environment, as well as where and when these materials will be released. This allows to connect buyers and suppliers of secondary materials, and to optimize the supporting logistics and storage via material hubs.
Street-level images and Artificial Intelligence
VITO has many years of experience in sustainable materials management on the one hand and data processing and remote sensing on the other. In the Demolition Guide project we used both areas of expertise and developed a proof-of-concept for automatic material recognition using street-level images and Artificial Intelligence with the aim of making a better and faster estimate of our material stock.
The generic workflow is independent of the materials or building elements to be recognized. We aimed to develop an end-to-end processing chain, from the street-level images as input data to an estimated value for an identified building element as output.
In this demonstration project we focused on detecting and delineating windows using Google Street View images. In addition, we analyzed the building façade altogether to determine a relevant building typology related to its materials content. This generic structure ensures that the workflow can be used or expanded further in similar tasks and follow-up projects.
The Demolition Guide generic workflow
The Demolition Guide workflow contains several modules:
-
Building image generator:
In a first module, we generate the images and metadata of the buildings that need to be analyzed. We use the official developer API of Google Street View to generate images based on location, heading, pitch and zoom levels of a façade. Based on the geographic data that we retrieve from the Large-Scale Reference File (GRB) Flanders, i.e. 3D LOD1 building data, we can determine the distance and the point of view with respect to the building façade and thus set the optimal parameters for further processing. -
Deep learning algorithms for window detection and building typology:
Building elements and typologies are estimated by applying deep learning algorithms (AI). For the Demolition Guide project, we focused on windows because of their wide application in the context of the circular economy: the possible reuse by real estate developers, recycling possibilities of the materials by window manufacturers, estimation of the energy efficiency of the building, ...
In addition, three building typologies are automatically recognized: brick, hidden brick, and residential versus non-residential buildings. The building typology allows to estimate the average material balance of the entire building. -
3D reconstruction module:
In this module we convert the pixel coordinates of the window vertices to 3D metric coordinates, hence the size of the window area can be estimated. -
Residual Value Estimator:
During this project, we have been investigating the relevance of using machine learning for estimating residual values of used building elements (i.e. windows) and materials. Novel data streams such as web scraping have been explored. Demand prices of used windows offered on online trading platforms have been logged. But also all corresponding specifications of those windows were used to determine the drivers for price-setting.
Printscreen of the demonstration tool showing the automatic material recognition of a building in Leuven.
How well does it work in practice?
After development, the entire workflow was, of course, tested. We applied automatic material recognition to several buildings in the city of Leuven (Belgium) and released the results to the stakeholders via an interactive map or demonstration tool.
Demonstration of the interactive map
We started from a pre-trained window detection model available in the international literature. With this we already achieve an average accuracy of 85% for an individual street scene, which could even be increased if we further refine the algorithm with training data for typical architectural styles in Flanders. In addition, the redundant detection from different points of view ensures that building elements missed in one image can still be captured from a different angle.
The three AI models for building typology recognition (“brick”, “hidden brick”, and “residential/non-residential”) were trained on our own generated dataset of several hundred facades in a number of Flemish cities. The achieved accuracy is above 90% for all models, with a score of 95% for brick detection as the best performing model.
“As a real estate project manager and sustainability advisor, we guide our clients in every project to the optimum between the economic, ecological and social aspects. The Demolition Guide can help us by quantifying and valorizing the residual value of materials in an efficient manner.”
Benjamin Huygels - BOPRO
What's next?
Demolition guide thus provided a first proof-of-concept for detecting building elements and materials on street-level images of buildings in Flanders, and it also provided us with some interesting technical insights to further improve and scale up our services.
- Scaling up to neighborhood or city level:
In this demonstration project we solely used Google street view images for building material detection. The main advantage is that the data is publicly available, but they also have a number of limitations, such as limited GPS accuracy, obstacles that make facades more difficult to see such as trees and cars, the update frequency, etc. However, this doesn't limit the possibility to scale the technology to neighborhood or city level on a relatively short term, which can significantly improve the currently available statistical data on the material stock within a city and how it will evolve in the future. - Increase accuracy and update frequency:
If we would refine the window detection algorithm with training data for typical architectural styles present in the region of interest, we could even further improve the recognition accuracy for an individual street image. Furthermore, the accuracy and update frequency can be improved with frequent mobile mapping data acquisition from transport vehicles, the use of advanced sensors (multispectral, thermal, LIDAR), and complemented with information extraction from aerial images and satellite data. - Explore novel data streams:
Further development of a residual value estimator for used materials by exploring more novel data streams (web scraping, sensors,…) and hence better understand the price setting mechanism.
-----------------------------------
This project was funded by OVAM – Flanders Circular and carried out by VITO Remote Sensing and VITO Materials in collaboration with partner Immoterrae. We thank the stakeholders in this project, City of Leuven, BOPRO, Tracimat and FLOOW2, for their valuable contribution from the end-user perspective.
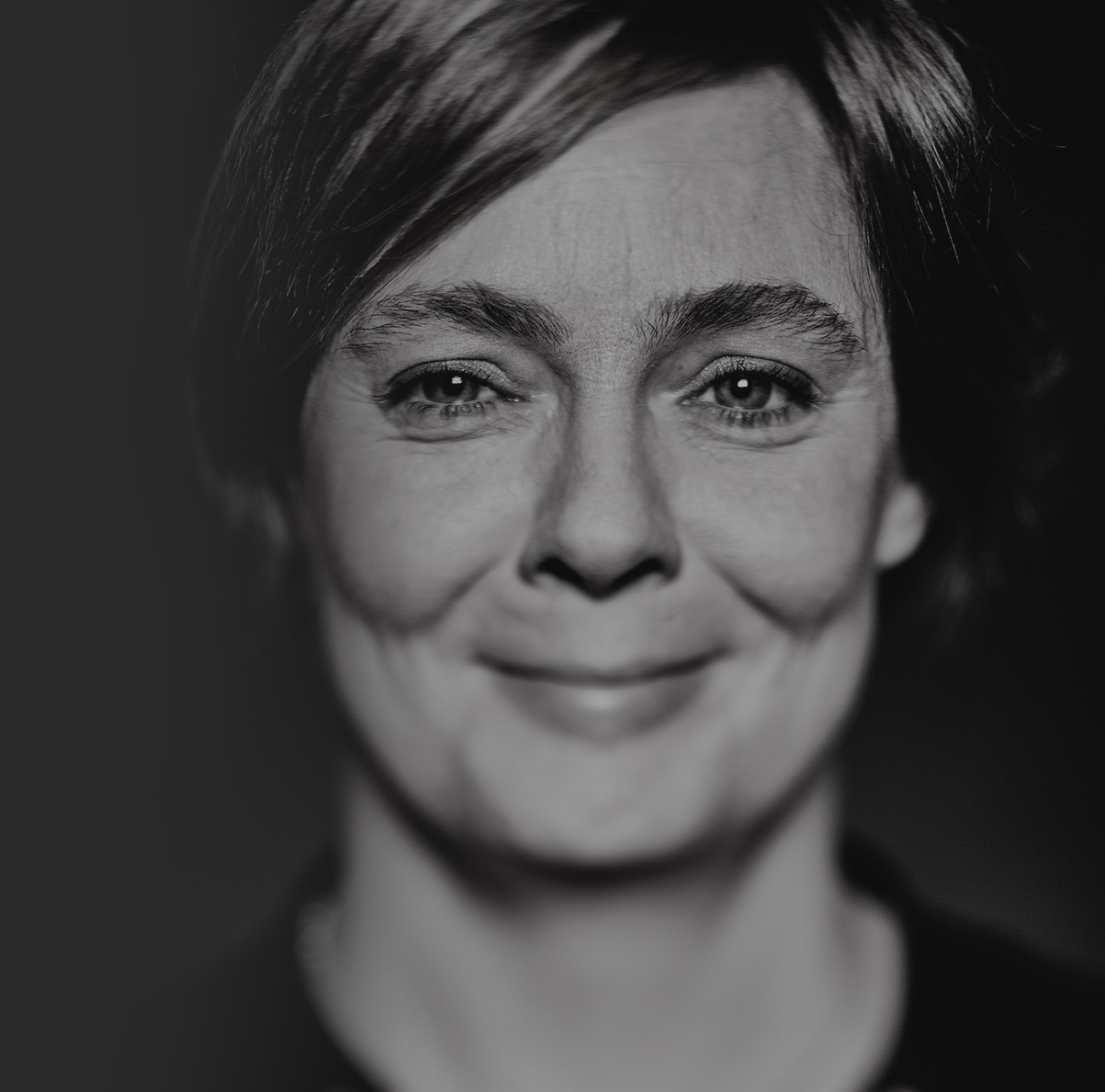