How to contain the spreading of the Pine Wilt Nematode towards Europe
The Portuguese Pine forest industry represents more than 2 % of the national GDP and is responsible for more than 50 000 jobs in over 8 000 companies. However, the last decennia, this growing industry is strongly affected by two main phenomena: forest fires and the (fast) spreading of the Bursaphelenchus xylophilus, commonly named the Pine Wilt Nematode (PWN), that emerges in Europe since 1999.
The PWN kills the Pine trees in less than a season and makes the tree timber unusable for the forest industry. In addition, the Nematode is spread from one tree to another one by a flying insect, the Monochamus Galloprovincialis, making it impossible to predict which tree(s) will exactly be the next victim(s) of the Nematode as the insect can fly several hundreds of meter before stopping on the following tree.
The only way to contain the spreading of the disease is to cut the hosting trees as soon as possible and to burn it directly on the field.
However, once the symptoms appear visually (yellowing of the needles), it is mostly too late as the contamination of the surrounding trees has probably already occurred.
A precise and detailed early detection of each individual contaminated pine tree is a pre-requisite to contain the disease. Fortunately, remote sensing techniques offer a reliable, efficient ànd affordable solution!
Fig. 1 :
left: 2019: the algorithm detects 3 trees marked as infected, one of them being marked as 'suspicious' as no symptoms are visible at that moment.
right: 2020: the 3 trees are dead, confirming the early detection performed in 2019.
Using COTS and Cheap technology to acquire the requested data
In order to offer an affordable operational solution, Cost-Of-The-Shelf (COTS) and easy to use technology must be preferred to complex, fragile and expensive scientific instrumentation.
After a thorough experimental study, performed in the framework of the H2020 FOCUS project (2018-2021), we succeeded to develop a system combining multispectral very high resolution (5-20 cm GSD) drone imagery (from a 5 bands Micasense Red-Edge M camera mounted on different types of 'low cost' drones, like e.g. the DJI Phantom Pro 4) with Machine Learning (Random Forest) algorithms.
Besides its very friendly use on the field, this solution also offers the advantage to have spectral bands very close to the ones offered by the Copernicus Sentinel-2 satellite system that can cover much larger areas. Of course with a significantly lower spatial resolution (10 m) compared to the drone images.
MAPEO Forestry: an operational service to support forest managers
The early detection of the pine trees affected by the PWN is a complex process. Based on an extensive literature study and on the analysis of the different flying campaigns performed during the FOCUS project (2 hyperspectral airborne campaigns and 6 multi and hyperspectral drone campaigns, performed during 3 years on 5 different locations in Portugal), we succeeded to identify 13 different indices, which combination allows us to detect suffering pine trees.
Moreover, the developed algorithm, using Random Forest Machine Learning techniques, allows to discriminate the exact status of the affected trees and classify them into 3 categories:
- suspicious trees (identified by the algorithm while no visual symptoms are present)
- contaminated trees (identified by the algorithm and presenting clear visual symptoms)
- dead trees (trees that no longer have needles)
Validation was performed using ground true data provide by the University of Coimbra.
The complete data processing has been fully automated and the user only needs to provide the raw data (multispectral images and references targets).
During the automated processing, several basic (level 1) products are created:
- a high resolution orthorectified image of the Region of Interest (RoI)
- a high resolution Digital Terrain Model (DTM) of the area
- a high resolution Digital Surface Model (DSM) of the area
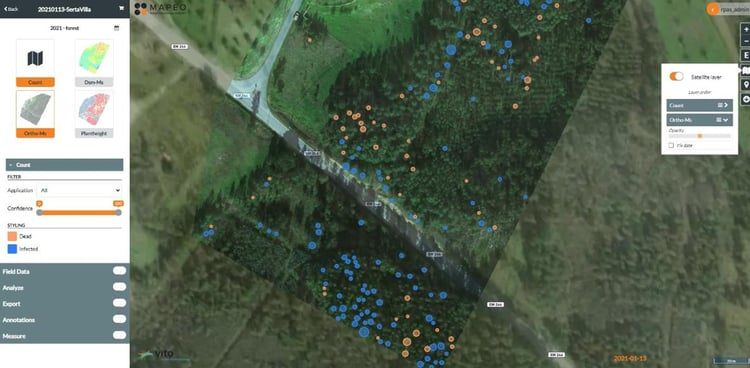
Fig. 2: Screenshot of the MAPEO application tool: orange spots correspond to dead trees, blue ones to infected trees.
The final generated products (level 2) are a high resolution orthorectified map where each individual affected tree is marked with different colors, according to their status (suspicious, contaminated, dead) and a list containing the tree number, its exact GPS position and its status.
Several intermediate products are also generated and can be delivered if required by the client.
Fig. 3: Zoom on a picture after final classification: red, blue and yellow dots correspond to respectively dead, infected and suspicious trees.
The MAPEO Forestry system is currently in a pre-operational phase (Beta version) and is ready to be validated by operational users. A GIS web interface allowing the visualization, annotations, definition of RoI, measurements as well as some statistics, has been customized to allow the users to analyze the results in an easy way and to export them in their systems. Standard archiving in the cloud is also foreseen.
WHAT'S NEXT?
We will anticipate on further customization of the GIS web interface in order to allow the user to change color and formats of the generated products.
If requested by our clients, we'll implement an automated generation of standard reports (containing e.g. basic statistics).
We'll keep you posted!
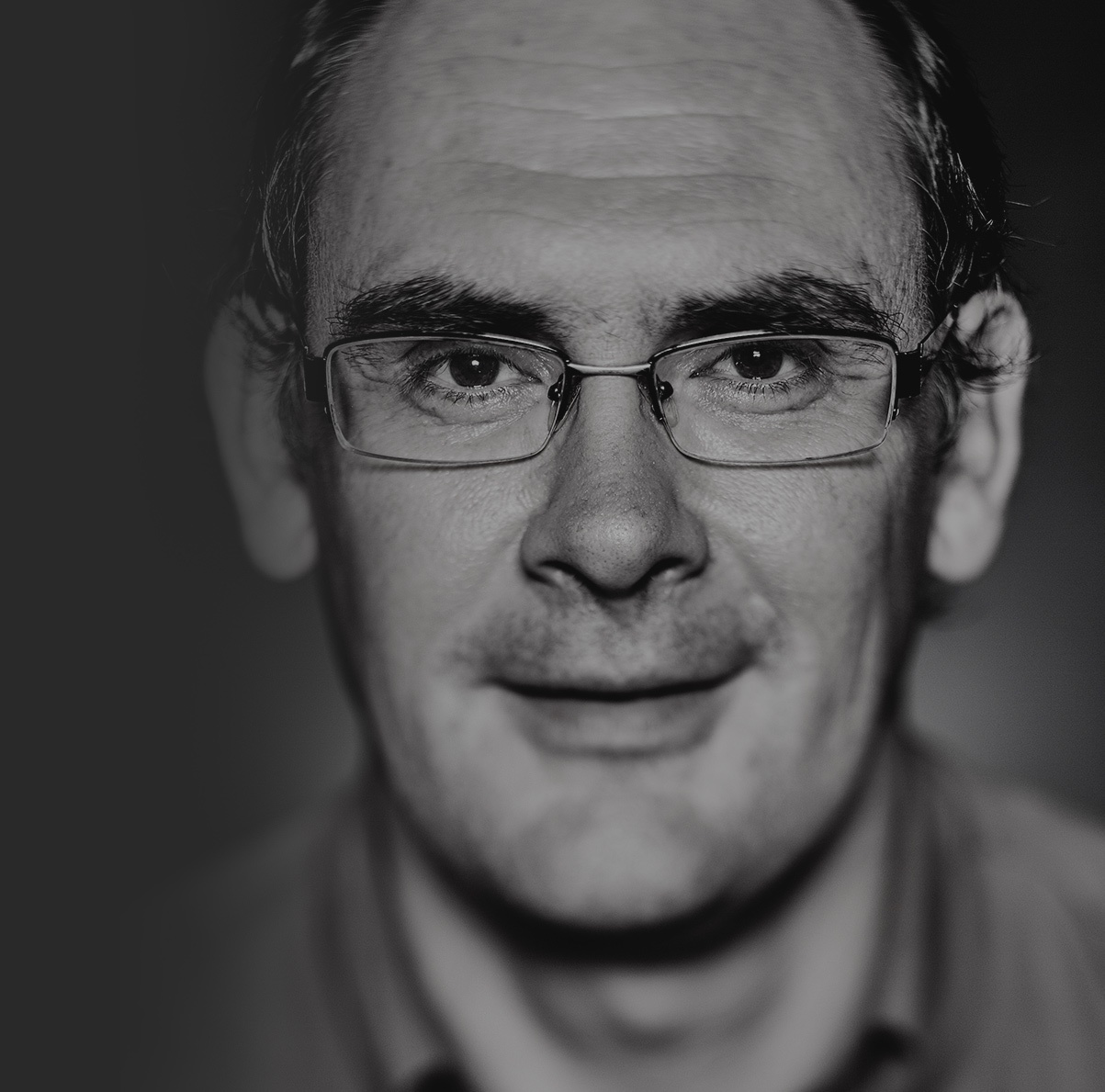