Deep learning for unsupervised land cover change detection
Intensified monitoring of land cover and land use is crucial to improve studies of forest loss dynamics and drivers, but also support policies related to agriculture, climate change mitigation, urbanization or biodiversity. RapidAI4EO brought together industry leaders including Planet, Vision Impulse, IIASA and Serco Italia to advance the state-of-the-art in Land Use Land Cover Classification and Change Detection from remote sensing observations. Within this project, VITO was responsible to develop (i) an unsupervised change detection algorithm based on Deep Learning that allows to identify hotspots of change and (ii) a land cover classification workflow to produce monthly land cover maps over these hotspots. These algorithms were developed using high resolution (i.e. 3 m Planet Fusion and 10 m Sentinel-2 data) and high cadence data (i.e. monthly updates).
One of the challenges that we faced when detecting changes using monthly satellite time series is that satellite images can present a lot of variation from one acquisition to another. For instance, phenological change - following the seasonality of vegetation - is present throughout the year, while altered meteorological conditions can lead to interannual change of the vegetation condition. All these changes cause a significant variation in the raw pixel values, yet they are not land cover changes.
Example of phenological change in a time series of Planet Fusion imagery of Sicily
This mixture of various change types can introduce irregular temporal dynamics of unchanged pixels and hence complicates the detection of real land cover change based on single pixel time series. The use of a long time span and the evaluation of contextual information present in the images may help to achieve a more reliable identification of land cover changes. Deep Neural Networks can for instance learn complex features from the images and encode the relevant (contextual) information into a mono-dimensional compressed vector which is easier to handle than the original images.
Seasonal versus structural changes
To this end, we developed an unsupervised change detection algorithm based on deep learning architectures to extract feature representations of image patches. The algorithm aims to identify areas whose land cover changed over time, and for which a land cover update at pixel level is thus needed.
We used the concept of triplet loss to extract compressed image features. We built upon the Tile2Vec algorithm that has originally been developed for learning compressed representations from unlabelled remote sensing data to classify images. Here, we adjusted the algorithm to ensure the separation of seasonal and structural information and hence facilitate the detection of land cover changes. We trained the algorithm on a set of 500 000 image Sentinel-2 and Planet Fusion image patches, where we explicitly rewarded the algorithm to minimize seasonal effects on the vector representation, while maximizing the distance between the vector representations due to a change in land cover.
Example of the change detection algorithm. The animation shows a rural area in Flanders containing forested areas, settlements and cropland. Although the land cover over the crop fields remains the same, the harvest and planting cycles cause a lot of variation over time. The algorithm has however learnt to ignore these changes and does not flag any change.
Example of the change detection algorithm. The animation shows a forested area in Portugal that faced forest loss. Three events in February 2019, June-July 2019 and June-July 2020 have been detected (the detected change is highlighted using a red square).
Challenges to provide monthly land cover classification
After identifying the patches that changed, we predicted monthly land cover at pixel level at 3 m (Planet Fusion) and 10 m (Sentinel-2) resolution.
Increasing the cadence of the land cover updates from (multi-)annual to monthly cadence however poses several challenges. First, several land cover types are difficult to discriminate without any knowledge of intra-annual temporal dynamics. For instance, croplands are characterized by a dynamic of vegetation growth and a harvest period (i.e. cycles of bare soil, sparsely vegetated and vegetated periods). This contrasts with grasslands that often lack the harvest period. Without this temporal information, it is difficult to distinguish a vegetated cropland field from grassland. Second, as aforementioned, phenological changes may introduce a large intra-class variability and thus also confusion between classes. For example, the shedding of leaves during the autumn-winter period or wilting of herbaceous vegetation in dry summer periods introduces spectral variability within land cover classes.
To overcome these challenges, we developed within RapidAI4EO a workflow with two classification phases: first we classified land surface characteristics using single observations subsequently followed by a land cover classification phase where we used one year of land surface characteristics as input. We applied the workflow over three areas of interest (within Belgium, Portugal, and Sicily) using Sentinel-2 and Planet Fusion time series over the years 2018-2020. Below you can see some examples of the resulting monthly land cover maps.
Example of land cover maps over Portugal using Planet Fusion (top) and Sentinel-2 (bottom).
Towards improved land cover monitoring
The detection of hotspots of change may contribute to an increased efficiency of the Copernicus Land Service. If the change detection algorithm is capable of identifying the areas that likely changed, the land cover classification algorithm should only update the land cover of those areas that were flagged as changed. This reduces the amount of data that needs to be processed by the classification algorithm. In addition, the project contributed towards the development of land cover maps at an increased temporal (monthly) and spatial resolution (3 m and 10 m resolution).
This project has received funding from the European Union’s Horizon 2020 research and innovation programme under grant agreement No 101004356. The RapidAI4EO partners consist of Planet, VITO, Vision Impulse, IIASA, and Serco Italia S.p.A.
Copyright images:
Header image: Copernicus Sentinel-2 mission takes us over palm oil plantations in East Kalimantan - the Indonesian part of the island Borneo - © contains modified Copernicus Sentinel data (2019), processed by ESA, CC BY-SA 3.0 IGO
Body images/animations: © RapidAI4EO
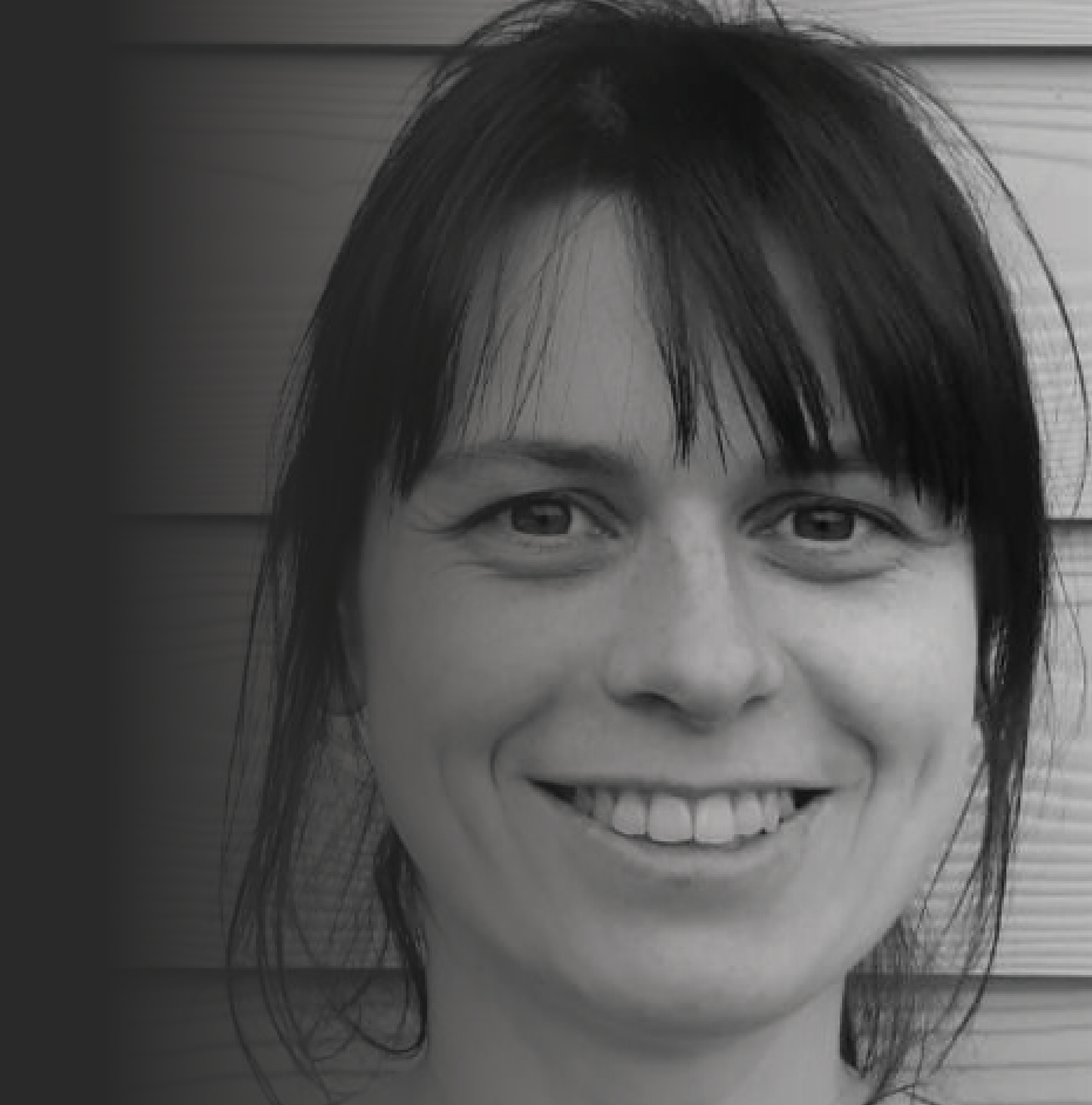