Deep learning for environmental policy support
Monitoring and protecting our environment is crucial for both the planets and people’s health. Satellite data and image processing techniques have an enormous potential to support environmental policy makers, but they are unfortunately not yet used to their full extent. The Geo.Informed project, funded by Research Foundation Flanders (FWO), aims to bridge this gap by leveraging machine and deep learning to maximize the value openly available satellite imagery in support of environmental policy makers in Flanders. Together with KU Leuven, the Institute for Forest and Nature Research Flanders (INBO) and Digital Flanders (team EODaS), our AI and environmental monitoring teams developed workflows using satellite imagery that meet policy makers’ needs.
Based on the needs that were stressed by governmental agencies during an interactive workshop, eight use cases were selected in which remote sensing data could support the policy decision process.
Consistent surface water monitoring using satellite imagery
INBO is responsible for monitoring biodiversity in Flanders. They manage a dataset of the permanent stagnant surface waters in Flanders, as their distribution is a key indicator for aquatic biodiversity. This is done based on visual interpretation of the aerial imagery that is acquired annually over Flanders, a very labor-intensive task. Moreover, a single observation does not allow any conclusions on hydroperiod. To improve this process, we aimed to use satellite imagery to (i) facilitate the dataset maintenance and (ii) provide insight on surface water seasonality.
The results of the Geo.Informed project show us that we can improve our efficiency in updating the dataset of permanent stagnant waters in Flanders. By using satellite imagery and remote sensing technology, we can not only streamline the maintenance process but also gain valuable insights into surface water seasonality.
An Leyssen - Research Institute for Nature and Forest (INBO)
The Copernicus Sentinel-1 and Sentinel-2 satellites have complementary strengths for surface water monitoring. Sentinel-2 optical imagery provides a clearer water signal and more spatial detail, but observations are hampered by clouds. Sentinel-1 provides consistent, weather-independent radar data, though it can sometimes misclassify water-like features. By combining both in a modular framework, water delineations are created from individual scenes and then aggregated into monthly products. This approach keeps data volumes manageable when focusing on permanent water bodies, while still allowing detection of temporary waters.
Overview of the modular framework, comprising a U-Net architecture applied on stacked Sentinel-1 and Sentinel-2 scenes and a CatBoost model to aggregate scene-based delineations into monthly products.
A U-Net architecture is used to provide a delineation of the water surface based on individual Sentinel-1 and Sentinel-2 scenes. By combining both and including clouds in the training dataset, the model learns to focus on the Sentinel-1 features when the Sentinel-2 scene is (partially) clouded. More details are provided in this paper.
Next, the scene-based delineations are aggregated into monthly products using a CatBoost model. This approach reduces both the uncertainty and the volume of data that end users need to process.
Scene-based predictions for the area around the Schulensmeer lake throughout 2023, a region and year unseen during model training.
Supporting water quality monitoring in Flanders
Another use case that could benefit using satellite data and remote sensing technology is water quality monitoring. Algae are a crucial part of the aquatic food chain. However, they can have a harmful effect when too abundant, and toxic species like cyanobacteria or blue-green algae exist. Together with the Flanders Environment Agency (VMM), responsible for monitoring biological quality elements like phytoplankton and reporting on surface water quality for the Water Framework Directive, we checked how satellite data can support their phytoplankton monitoring process. Currently, they perform monthly field campaigns in a selection of water bodies. By leveraging remote sensing, they can gain insight in the frequency, duration and spatial coverage of algae blooms, which unlocks additional possibilities for water quality assessments.
Due to a lack of reference data, we focused on a validation of existing algorithms to retrieve chl-a concentrations. We processed and filtered over 1.5 million measurements from various institutes based on their proximity to the nearest Sentinel-2 image, resulting in 1,150 matchups. We assessed algorithms exploiting the blue and green bands, the red and near-infrared (NIR) bands as well as algorithms that consider a larger part of the Sentinel-2 spectrum. From these, the Blend switching algorithm and Mixed Density Network (MDN) algorithm demonstrated the best performance. More details can be found in this paper. The MDN algorithm is already used to create chl-a products over Belgium in near-real time. The products are available via the Terrascope platform.
Screenshot of the chl-a product, provided in near-real time through the Terrascope viewer.
A user-friendly web viewer
Developing advanced machine learning methods is essential, but we also need to check data access and integration into the users’ workflows. To support this, we created a user-friendly web viewer that enables policy makers to easily access objective insights and seamlessly incorporate them into their daily decision-making processes. The results from the surface water monitoring use case, along with three others primary use cases, are made available through this interactive web viewer. It not only shows the model outputs, but also incorporates additional data sets such as reference datasets, topography, aerial and Sentinel imagery, to facilitate interpretation of the model outputs.
Demonstration of the Geo.Informed interactive web viewer
No model is perfect, so it’s crucial to consider uncertainty when using model outputs for decision making. To address this, users can switch between the overview viewing mode, where each pixel gets the color of the most probable class, and the single class mode. When selecting a single class, a threshold slider is activated. Only pixels with a model probability above this threshold for the selected class are now visualized. Users can thus interactively make the model outcome more conservative, by increasing the threshold, or can also include less certain detections, by lowering the threshold.
Finally, the viewer also encourages stakeholders contribution for future model development. Stakeholders know their use cases so they are best suited to detect errors and biases. They can provide feedback by drawing annotations, which are automatically saved and can be used to set up active learning frameworks and continuously improve the models in the future. Together with the stakeholders, Digital Flanders (EODaS) aims to further explore how the project results (data, models, and viewer) can be further utilized in the future.
Interested in the Geo.Informed web viewer, surface water or water quality monitoring? Contact us!
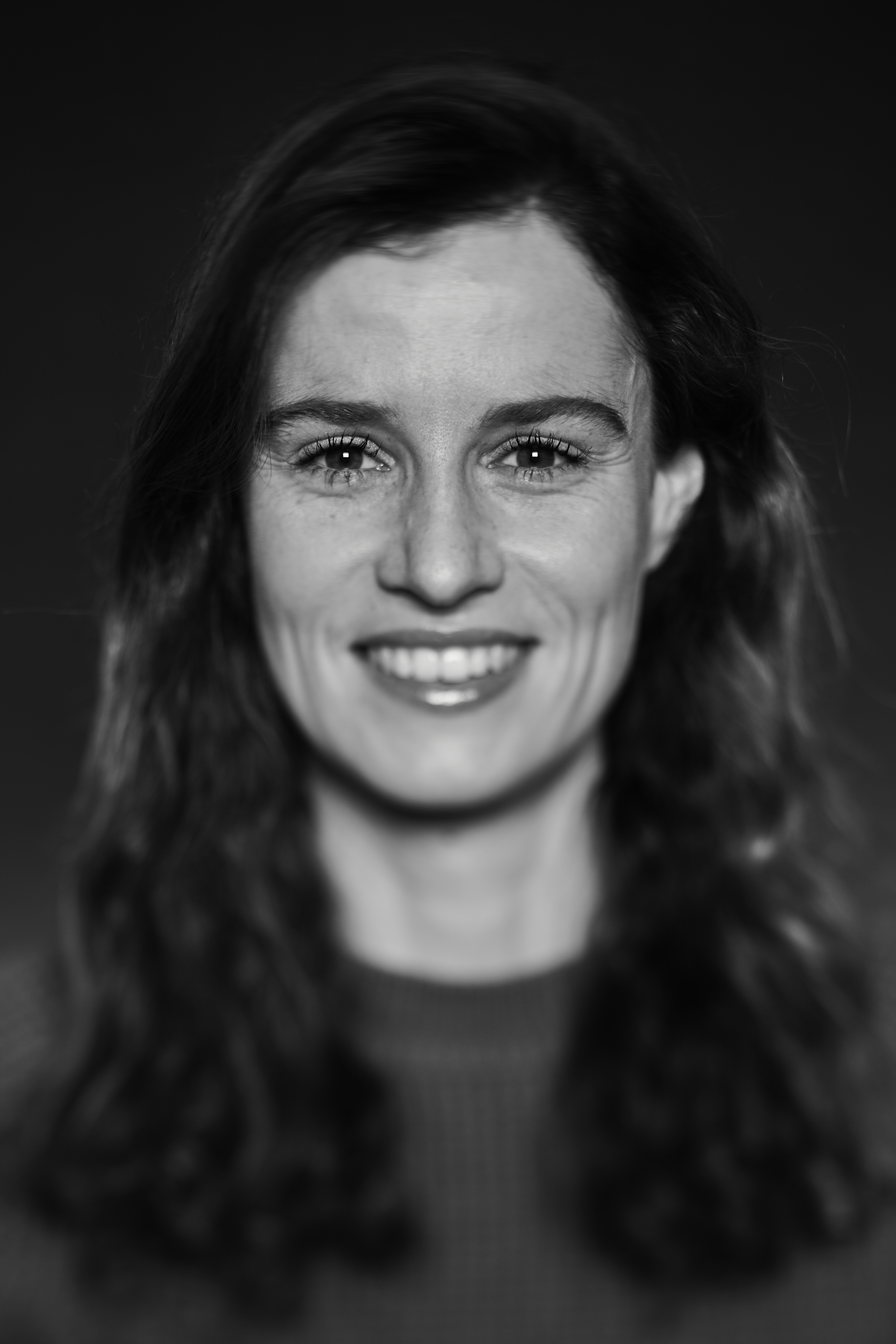