Evolution in targeting accurate and scalable habitat maps
With our global landscape under pressure and ambitious targets set to conserve and restore our ecosystems, we feel the need to further improve habitat mapping to support biodiversity and ecosystem management. In 2022, we obtained first results in the use of AI to create habitat maps that show actual habitats at a certain moment in time. Even though these habitat maps often serve as intermediate products in the ecosystem extent accounting workflow, they also contain crucial information about the Red List status linked to many habitats. This information can contribute to the reporting of Goal A.2 (extent of natural ecosystems) the Global Biodiversity Framework and guide landscape planning and inform conservation or restoration efforts.
Initially, our habitat mapping workflow used public training data from the European Vegetation Archive (EVA). Now, we enhanced it with national field inventory plot records, addressing the EVA's limitations in plot availability and diversity in regions like Scandinavia, the Azores, and sparse vegetation habitats. These field-validated plots improve the geolocation accuracy and increase the number of training points per class, as EVA plots often vary in geolocation reliability. Additionally, the workflow now supports additional predictors, such as high-resolution vegetation height, and performs comprehensive hierarchical classification for EUNIS level-2 and level-3 classes. We've also developed a new post-processing module that adjusts habitat classifications per pixel, reconciling differences between model predictions and reference maps like CLC+ Backbone.
Demonstrating innovative methods for trustworthy results
The enhanced habitat mapping workflow, initially based on the work done in EuropBON, was demonstrated in the PEOPLE-EA project in Slovakia, amongst other countries.
Visual scheme of the hierarchical Machine Learning and AI habitat mapping workflow.
Local training data is gathered to obtain a field-validated collection of geolocation linked to a EUNIS habitat class. The EUNIS habitat classification system harmonizes habitat classification across Europe, facilitating easier reporting at the European level. This hierarchical system (up to 6 levels) covers all habitat types, from natural to artificial. We rely on national authorities to provide training data up to EUNIS level 3, trusting local fieldworkers' expertise to deliver comprehensive geospatial input covering all EUNIS classes in the region. Often, this requires the translation of the national habitat classification to the EUNIS classification.
Collected training point locations with associated EUNIS level 1 habitat class to conduct habitat mapping workflow for Slovakia, Stredné Slovensko region.
For the locations of the training data, we extract the corresponding values for all features in the feature set, which includes Earth observation data (Sentinel-1 and Sentinel-2 band reflectances and derived indicators), LiDAR or DEM data, soil data, climate data and spatial context. The combined set of responses per feature is then linked to the training datapoints and their habitat class. The habitat mapping workflow is a hierarchical tree:
- first EUNIS Level 1 classes are identified
- followed by Level 2 classes within Level 1 borders
- and finally Level 3 classes within Level 2 categories
The branches of the tree represent specific models to map EUNIS sub-classes within the higher level. Within one model, we select the best predictors to distinguish different classes based on a separability and redundancy analysis. These predictors and the training data are used to train that particular AI/ML model, a gradient boosting framework on decision trees (CatBoost). This model is then applied across the entire area of interest, selecting one discrete habitat class per pixel to create a comprehensive map of EUNIS habitat classes. The CatBoost model output also includes classification probabilities per class. These probability maps can be revised in a post-processing step; if the highest-probability class does not match with reference maps (e.g., CLC+ BackBone or MaxEnt suitability maps), the second-highest probability class might be a better option, allowing pixel-level habitat class corrections.
Hierarchical habitat mapping approach: EUNIS Level 1 – EUNIS Level 2 – EUNIS Level 3
The hierarchical modelling approach allows consistent and more trustworthy results. Given the importance of the initial Level 1 classification (rf. The hierarchical modelling approach), we also generate 'confidence maps' indicating whether pixel classifications have been altered due to mismatches or matches with the CLC+ BB class.
The interaction between remote sensing and field validation
Consistently and efficiently evaluating the accuracy of created habitat maps remains challenging due to the lack of validation habitat maps in the study area. For Slovakia, a visual check of our habitat map against the national map showed better landscape detail capture compared to the national map, using Google Satellite imagery as a base. Although our Remote Sensing workflow can improve the process and quality of mapping habitats, the EO-based approach cannot function without fieldwork. Validation of results in the field is essential and reciprocal. The workflow depends on accurate field-validated datapoints for training data; the better the training dataset, the better the habitat mapping outcome. Local field experts also play a key role in validation. Conversely, fieldwork benefits from the RS approach, which optimizes time and cost efficiency and guides field interventions in priority areas through continuous monitoring.
Comparison between national habitat map of Stredné Slovensko with habitat map produced by VITO’s ML/AI-based workflow, on EUNIS Level 3.
Towards comparable habitat and extent maps on a global scale
With the extensive experience we’ve gathered over the last years and the new flexible workflow to adapt our habitat maps for various regions, we can now take the next steps to scale this method effectively to a European service. If an adequate number of training points for each EUNIS Level 3 category per environmental zone are accessible, the training database can be applied for habitat mapping in diverse areas within similar environmental zones, regardless of the origin of the data points.
In the upcoming WEED project, which recently started under authority of ESA, we aim to upscale our workflow to create European wall-to-wall habitat maps spanning 2016 to 2024, integrating a change detection method. We also plan to enhance workflow automation and user-friendliness by integrating it into the openEO platform. This will enable national authorities to autonomously run the workflow themselves, with a preliminary check on the sufficiency and quality of the provided training data, supporting their biodiversity and ecosystem management and assessment process. The workflow will also be tested beyond Europe, using public databases like TRY, BIEN, and GBIF to generate national vegetation maps.
Accurate habitat mapping at EUNIS Level 3 is a vital and promising field of research. These habitat maps are essential for deriving Ecosystem Extent maps, which UN member states are required to produce annually for amongst others ecosystem accounting and Global Biodiversity Framework reporting. A general cloud-based workflow providing global applicability with regional context is an interesting approach to create comparable habitat and extent maps on a global scale and supporting many decision-making processes.
Join us at the Love Tomorrow Conference to find out more or contact us to get in touch.
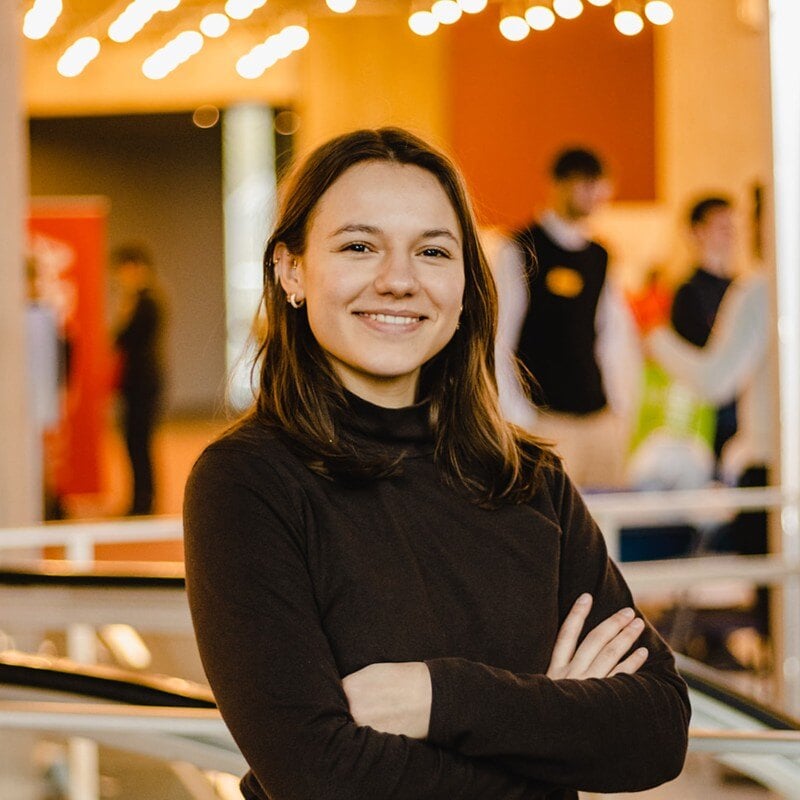